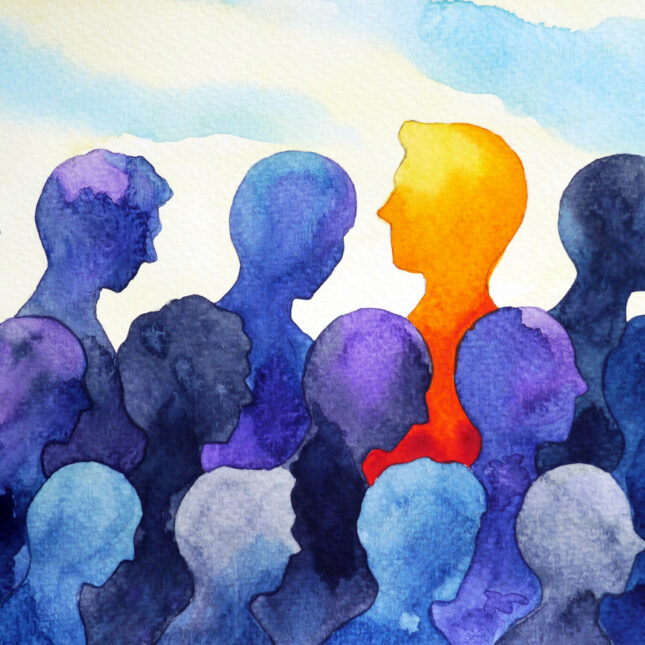
Vendors of electronic health records and other health technology platforms have begun to publicize and demonstrate “patients like mine” capabilities, which insert analytics distilled from EHR data into the physician workflow to guide clinical decisions. While these implementations could be helpful, simple analytics must not be passed off as evidence, and care must be taken to rigorously implement and vet these tools to avoid the negative clinical and cost outcomes associated with incorrect care decisions.
At its core, the “patients like mine” concept is simple: The outcomes of similar patients for each care choice being considered are made available to a health care provider, as if she or he asked “What happened to similar patients for whom the same choice was made?”
Vendors are exploring “patients like mine” as a way to evolve the EHR from its historically passive role in the clinical workflow toward a more active one. To date, the limited active roles for EHRs have centered mostly on alert-driven clinical decision support (CDS) that nudged or enforced providers to comply with guidelines or processes explicitly approved by the health system. This form of clinical decision support has been shown to broadly improve clinical outcomes and process quality. But when implemented incorrectly, it can also lead to disastrous consequences.
As a physician and informatics researcher, I have long believed in the tenets of evidence-based medicine established across the last half century, and have sought to build technology that integrates reliable precision evidence into the care provided for everyone. Yet with more and more of this work being driven by the tech and investment communities, I have grown increasingly concerned that the core methodological tenets the medical community relies on are being stepped over, creating risks for patients, unexpected costs to the health care system, and increasing liability risk for medical professionals.
The medical community’s process for generating and implementing evidence for care is well established and fueled by clinical trials, observational research, and meta-analyses backed by agreed-upon methodologies and peer review. Findings are summarized into guidelines, and extensive training reinforces health care professionals’ ability to identify trusted sources of evidence and use the best evidence available to them.
Despite the rigor and volume of standard clinical evidence, it alone is not enough to meet the needs that precision medicine requires. In some specialties, less than 20% of daily medical decisions are supported by quality evidence. Technology can play a critical role to rapidly create observational evidence sourced from similar patients and enable the use of that evidence at the bedside. Big tech and large EMR players have begun developing “patients like mine” technology and their PR machines are building excitement about the promising future.
Any evidence-providing technology, however, needs to ensure that the standards of transparency, data quality, and methodological rigor are being met. Regulations already hold life sciences companies, which also have large financial incentives in clinical evidence, to high standards when it comes to how they generate and communicate evidence for their products.
Automating “patients like mine” is risky and can cause harm
Let me pose a simple scenario that occurs thousands of times a day across the nation: a patient comes to their primary care physician with uncontrolled high blood pressure despite six months of attempted control on low-dose losartan, a common first-line drug in the angiotensin II receptor blocker class of blood pressure medicines. The EHR identifies 10,000 “similar” patients and displays for half a dozen possible blood pressure therapies their expected changes in blood pressure after six months along with their five-year heart attack risk. The physician sees that blood pressure appears best controlled with hydrochlorothiazide (another common blood pressure medicine in the class of thiazide diuretics), and the five-year heart attack risk is lowest by a few percentage points, and so adds it to the existing treatment regimen, and sends the patient on their way.
The addition does indeed help control the patient’s blood pressure. But 12 months later, the patient sees his doctor because of pain and swelling in his big toe. He is diagnosed with gout, a condition that thiazide diuretics increase the risk of. The patient now needs expensive chronic management for a painful and debilitating ailment that could have been avoided by taking a more evidence-based approach of either adjusting the dose of losartan or adding an alternate medication.
If recommendations like the scenario I described are built into clinical workflows, it will play out hundreds of times across the country.
What went wrong in that instance, and how can it be prevented?
First, the EHR automatically defined “similar” without the physician adding important clinical criteria for their patient. Because “similar” can be defined in any number of ways, understanding what is the physician asking is essential for correctly defining “similar.”
Second, patients can be “similar” at different points in their clinical journeys; making sure “similar” patients are at the same decision point is essential. In the hypertension scenario, a patient could have uncontrolled blood pressure any number of times over the course of their life and, if all such points are included, the most intense treatment regimen will likely show the best outcomes — but such a regimen will also likely come with the most adverse events. Using an appropriate methodology to identify the decision point, as well as to match patients on demographic and clinical characteristics related to their outcomes, is necessary to produce reliable clinical suggestions.
Third, by providing only simple analyses of the percentage of patients with controlled blood pressure or 5-year heart attack risk, and not confidence intervals around the point estimate, “patients like mine” fails to convey uncertainty in the prediction.
Fourth, these methodological issues are compounded by messy EHR data which should be cleaned prior to preforming predictive tasks.
Five steps to correctly get to evidence for “patients like mine”
To correctly generate evidence from observational data like that extracted from the EHR to guide therapy for “patients like mine,” the following criteria must be met:
Use proper statistical methodology. Appropriate statistical methodology that controls for confounders in observational data is essential to drawing conclusions from real-world data.
Standardize data quality evaluation. Datasets used for predictive purposes need to be cleaned for purpose and each time a cohort of patients is created for a “patients like mine” analysis the cohort needs to be assessed as being statistically powered to answer the clinical question being asked.
Standardize definitions of clinical concepts. A condition like diabetes can be defined in many different ways from looking at diagnostic codes, medications, lab values, and a combination of these over time. Definitions must be transparent to allow providers to know if their patient meets the criteria.
Regulatory grade transparency and auditability. Any recommendation made by a “patients like mine” system should be traceable, including source data, methods, and code used to implement the analysis. Such “patients like mine” tools are to be regulated as medical devices in guidance released by the FDA in late 2022.
Convey information and visualization to providers. Providers need to be given enough information to contextualize a recommendation and determine if their patient is appropriately represented in it.
While I remain excited and enthusiastic about the potential of bringing precision evidence to care for everyone, and believe that “patients like mine” approaches are an integral way to improve decision-making, I believe it is imperative to stick to sound methodology and transparency when generating evidence. Implementing dashboards with superficial analytics without understanding the underlying clinical scenario will lead to worse outcomes for patients and higher costs to an already overburdened health system.
Saurabh Gombar, M.D., Ph.D., is the chief medical officer of Atropos Health, which creates real-world evidence for health systems and life science companies, and an adjunct professor of medicine at Stanford School of Medicine.
LETTER TO THE EDITOR
Have an opinion on this essay? Submit a letter to the editor here.
To submit a correction request, please visit our Contact Us page.
STAT encourages you to share your voice. We welcome your commentary, criticism, and expertise on our subscriber-only platform, STAT+ Connect